Federated Learning for Sparse Principal Component Analysis
時 間:2023-10-25 14:00 (星期三) / 地 點:S101 / 茶 會:S205 (13:30)

李育杰
國立陽明交通大學 應用數學系教授
In the rapidly evolving realm of machine learning, algorithm effectiveness often faces limitations due to data quality and availability. Traditional approaches grapple with data sharing due to legal and privacy concerns. The federated learning framework addresses this challenge. Federated learning is a decentralized approach where model training occurs on client sides, preserving privacy by keeping data localized. Instead of sending raw data to a central server, only model updates are exchanged, enhancing data security. We apply this framework to Sparse Principal Component Analysis (SPCA) in this work. SPCA aims to attain sparse component loading while maximizing data variance for improved interpretability. We introduce a least squares approximation to original PCA, adding an l1 norm regularization term to enhance principal component sparsity, aiding variable identification and interpretability. The problem is formulated as a consensus optimization challenge and solved using the Alternating Direction Method of Multipliers (ADMM). Our extensive experiments involve both IID and non-IID random features across various data owners. Results on synthetic and public datasets affirm the efficacy of our federated SPCA approach.
個人網頁 https://www.citi.sinica.edu.tw/pages/yuh-jye/index_zh.html
相關資訊 https://aic.cgu.edu.tw/p/404-1044-102897.php?Lang=zh-tw
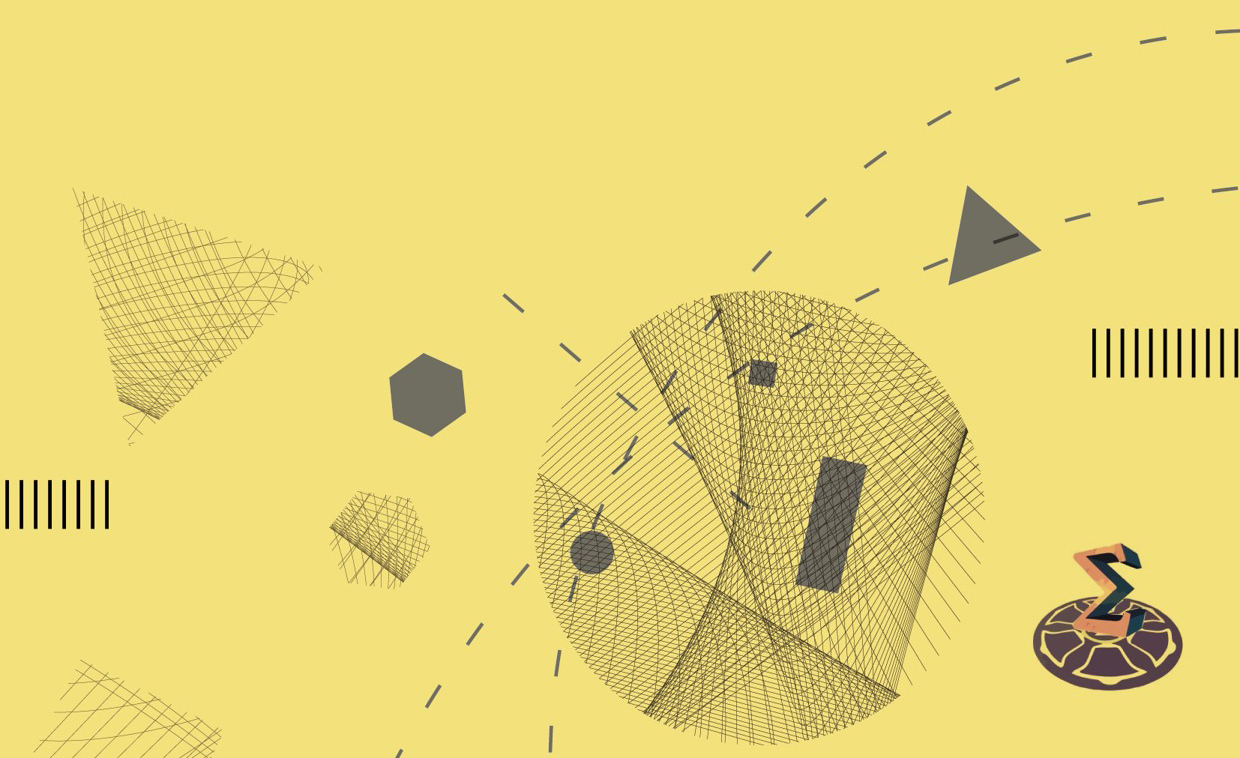