Novel and Tractable Convex Relaxations of Standard Quadratic Optimization Problems under Cardinality Constraints
Time: Apr. 8 (Tue.) 14:00-15:00
Place: M212, Mathematics Building, Gongguan Campus, NTNU
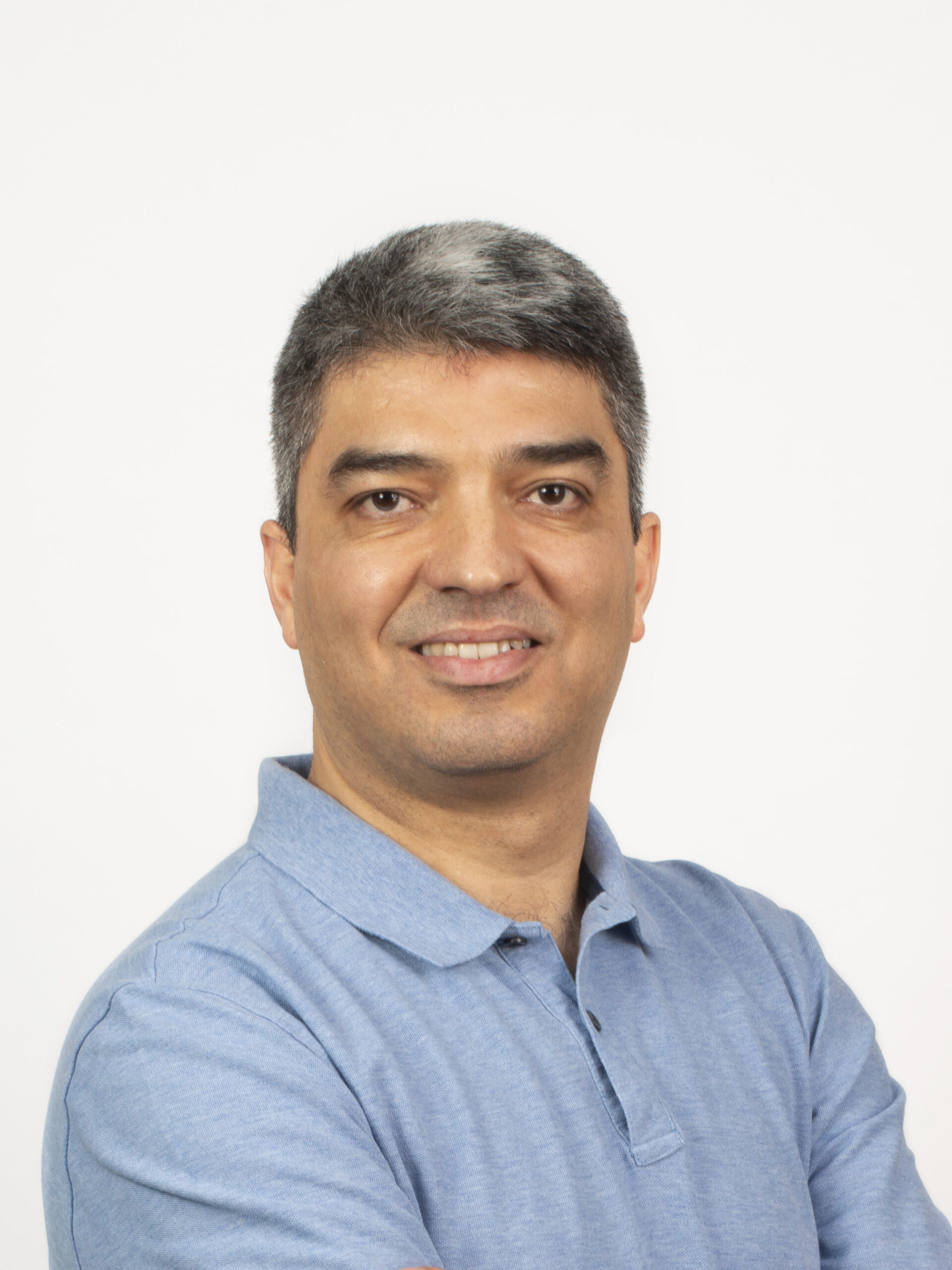
Alper Yildirim
Reader in Operational Research, School of Mathematics, The University of Edinburgh
Standard quadratic optimization problems (StQPs) provide a versatile modelling tool in a multitude of applications such as mathematical finance, machine learning (clustering) and modelling in biosciences (e.g., selection models and ecology). In this talk, we consider StQPs under an additional cardinality (sparsity) constraint which, even for convex objectives, renders NP-hard problems. One motivation to study StQPs under such sparsity restrictions is the high-dimensional portfolio selection problem with too many assets to handle, in particular in the presence of transaction costs. We present novel computational approaches to this relevant but difficult problem, involving modern conic optimization techniques, along with significant dimensional reduction, which is essential for tractability of these methods when problem size grows. In addition, we propose a particular generation procedure that systematically avoids too easy instances. We present extensive computational results demonstrating the versatility and strength of the proposed relaxations.
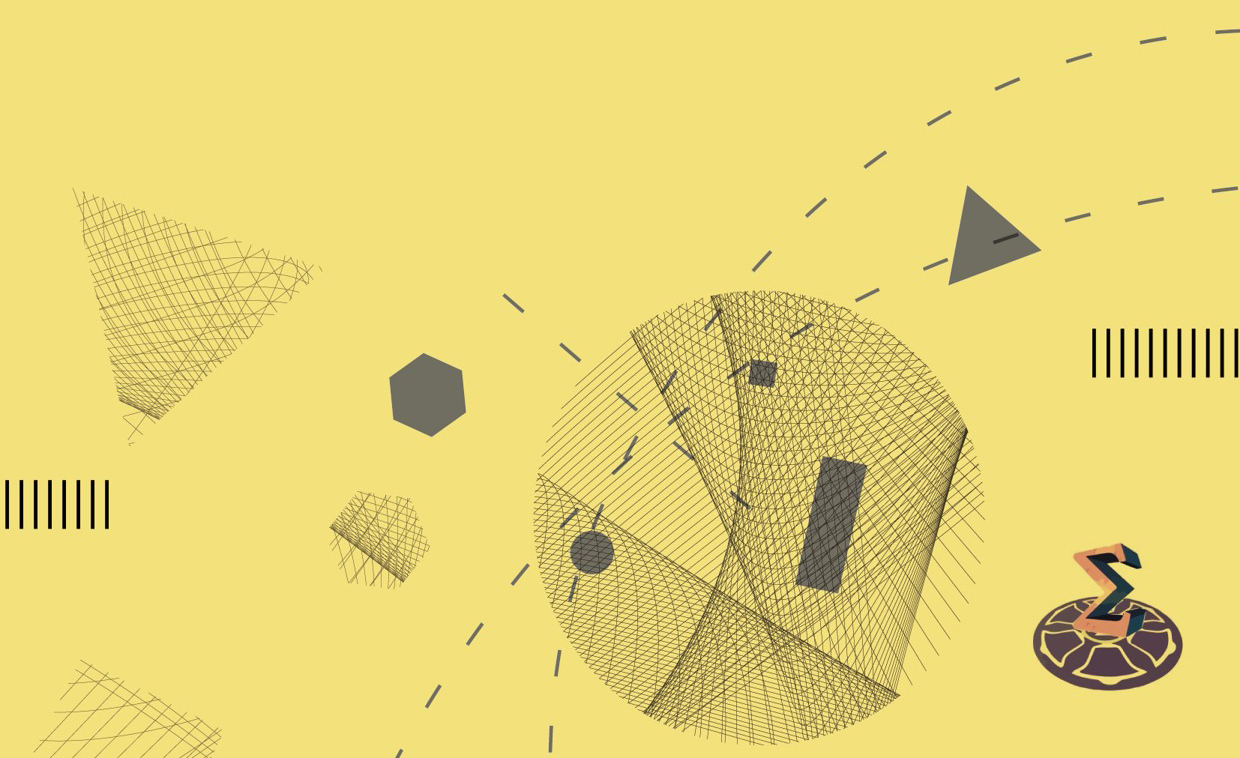